Introduction to AI-TRiSM (Trust, Risk & Security Management)
As AI reshapes the world, its transformative power drives revolutionary innovations across every sector. The benefits are immense, offering businesses a competitive edge and optimizing operations. However, to harness this potential responsibly, we must prioritize ethical and trustworthy AI development and usage.
This is where the concept of AI-TRiSM, a framework conceptualized by Gartner, emerges as a cornerstone for responsible AI development. It emphasizes three crucial concepts: Trust, Risk, and Security Management (TRiSM) in AI systems. By focusing on these key principles, AI TRiSM aims to build user confidence and ensure ethical and responsible use of technology that impacts everyone.
The Framework of AI TRISM
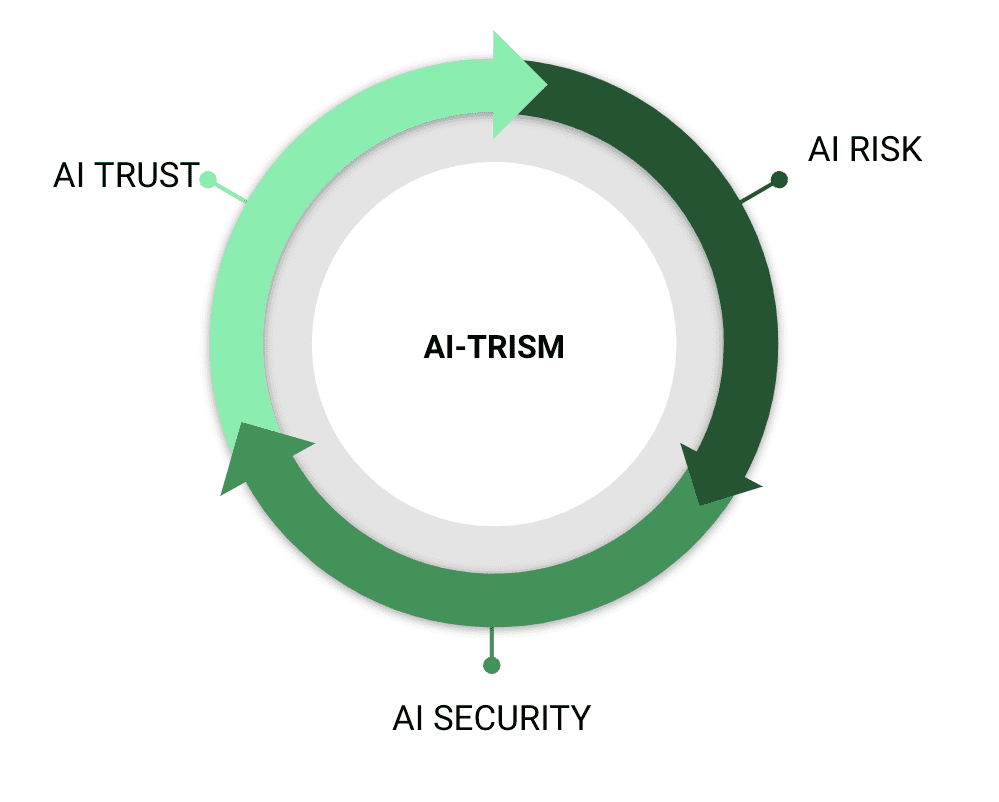
- 1. AI Trust: This cornerstone emphasizes transparency and explainability. By ensuring AI models provide clear explanations for their decisions, users gain confidence and trust in the technology.
- 2. AI Risk: This pillar focuses on mitigating potential risks associated with AI. By implementing strict governance practices, organizations can manage risks during development, deployment, and operation stages, ensuring compliance and integrity.
- 3. AI Security Management: This crucial aspect focuses on safeguarding AI models from unauthorized access, manipulation, and misuse. By integrating security measures throughout the entire AI lifecycle, organizations can protect their models, maintain data privacy, and foster operational stability.
4 Pillars of AI TRiSM Framework
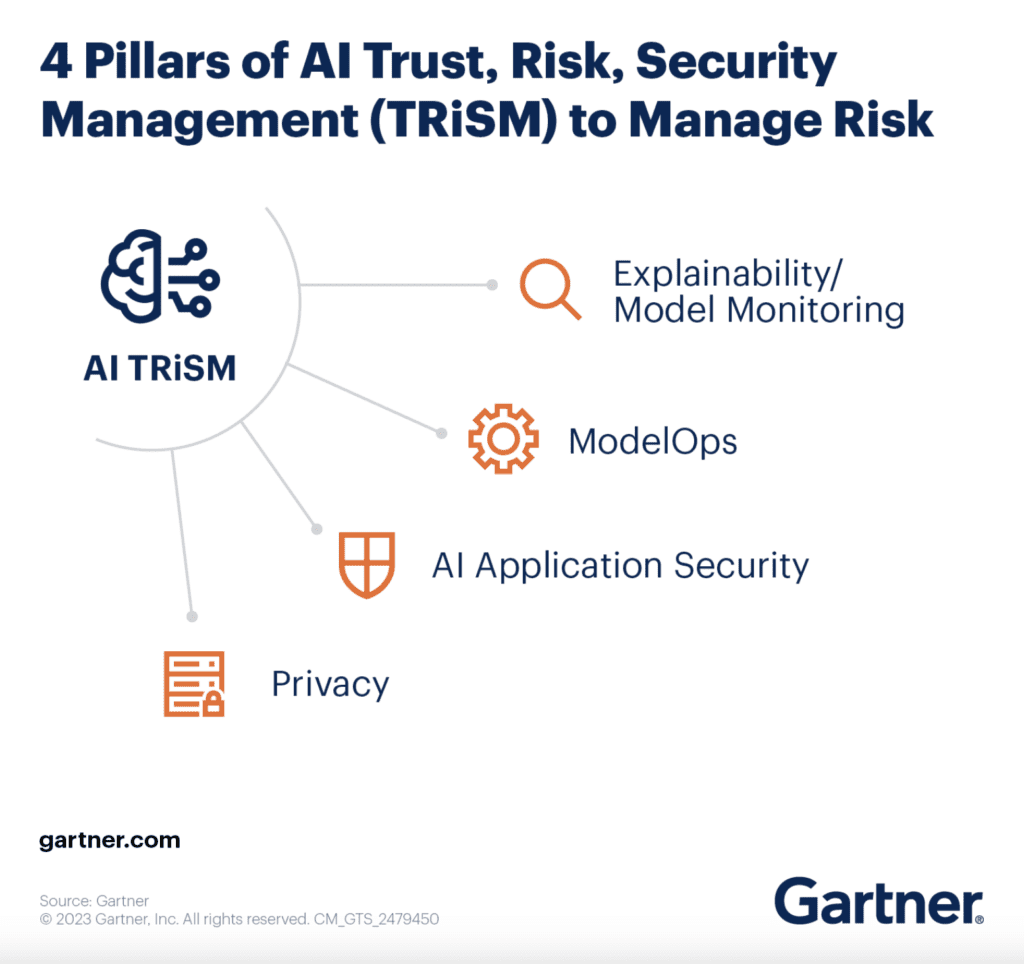
- 1. Explainability and Model Monitoring: This pillar combines two crucial elements. Explainability ensures transparency in how AI models arrive at their decisions, building user trust and facilitating performance improvement. Model monitoring involves continuously tracking the model's behavior, identifying any biases or issues impacting its accuracy and effectiveness.
- 2. ModelOps: This pillar focuses on establishing a well-defined lifecycle management for AI models. It encompasses the entire journey, from development and deployment to ongoing monitoring, maintenance, and updating. Robust ModelOps practices ensure the continued reliability and effectiveness of AI models over time.
- 3. AI Application Security: This pillar safeguards the integrity and functionality of AI models and their applications. It involves implementing security measures throughout the AI lifecycle to protect against unauthorized access, manipulation, and misuse. This ensures the reliability of the model's outputs and protects sensitive data.
- 4. Privacy: This pillar emphasizes the responsible handling of data used in AI models. It involves ensuring compliance with relevant data protection regulations and implementing appropriate security controls. By prioritizing data privacy, organizations can build user trust, minimize the risk of data breaches, and ensure responsible AI development and deployment.
Adopting AI TRiSM Methodology for Network CopilotTM
Network Copilot transcends the typical tech offering. It’s a conversational AI crafted to meet the complex demands of modern network infrastructures. Its design is LLM agnostic, ensuring seamless integration without disrupting your current systems, and doesn’t demand a PhD in data science to get started. Engineered with enterprise-grade compliance at its core, it offers not just power but also reliability and security.
Dive deeper today because with Network Copilot™, you’re getting seamless integration, enterprise-grade reliability, and enhanced security—all with ease
1. Documentation of AI Model and Monitoring:
2. Well-defined Life Cycle Management:
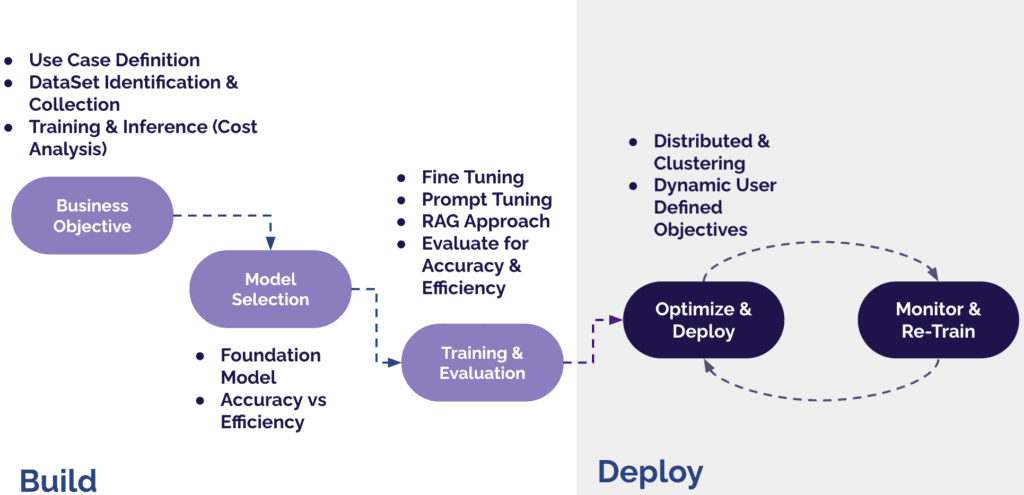
3. System Checks and Bias Balancing:
4. Responsible Handling of Data:
Conclusion
Start your Network Copilot journey: Contact us